Image search results used to give you the option to “view image” without having to navigate to the site the image was hosted on.
When it started in 2013, sites saw a 63% decline in organic traffic from image results.
Why?
Because there was no need to click through when the image could be viewed in full from within the search results.
And then everything changed
In February 2018, Google decided to remove the “view image” button. Now searchers must visit the site hosting that image directly, restoring image results to their former organic search driving power.
According to some recent studies, this change has increased organic image traffic a massive 37%.
Given image results’ return to value, marketers are asking themselves how they can make the most out of this search mechanism.
So what are some new ways we can leverage tools to better understand how to optimize images for ranking?
To explore this, I decided to see if Google’s Vision AI could assist in unearthing hidden information about what matters to image ranking. Specifically, I wondered what Google’s image topic modeling would reveal about the images that rank for individual keyword searches, as well as groups of thematically related keywords aggregated around a specific topic or niche.
Here’s what I did — and what I found.
A deep dive on “hunting gear”
I began by pulling out 10 to 15 top keywords in our niche. For this article, we chose “hunting gear” as a category and pulled high-intent, high-value, high-volume keywords. The keywords we selected were:
- Bow hunting gear
- Cheap hunting gear
- Coyote hunting gear
- Dans hunting gear
- Deer hunting gear
- Discount hunting gear
- Duck hunting gear
- Hunting gear
- Hunting rain gear
- Sitka hunting gear
- Turkey hunting gear
- Upland hunting gear
- Womens hunting gear
I then pulled the image results for the Top 50 ranking images for each of these keywords, yielding roughly ~650 images to give to Google’s image analysis API. I made sure to make note of the ranking position of each image in our data (this is important for later).
Learning from labels
The first, and perhaps most actionable, analysis the API can be used for is in labeling images. It utilizes state-of-the-art image recognition models to parse each image and return labels for everything within that image it can identify. Most images had between 4 and 10 identifiable objects contained within them. For the “hunting gear” related keywords listed above, this was the distribution of labels:
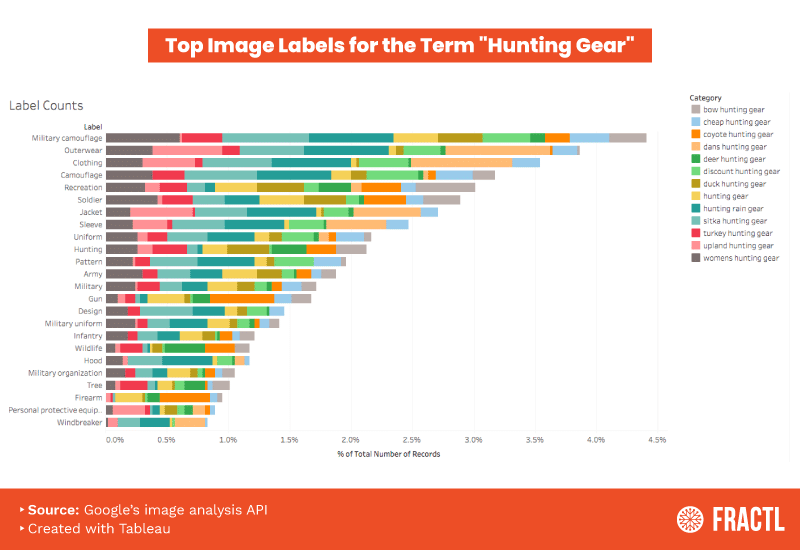
At a high level, this gives us plenty of information about Google’s understanding of what images that rank for these terms should depict. A few takeaways:
- The top ranking images across all 13 of these top keywords have a pretty even distribution across labels.
- Clothing, and specifically camouflage, are highly represented, with nearly 5% of all images containing camo-style clothing. Now, perhaps this seems obvious, but it’s instructive. Including images in your blog posts related to these hunting keywords with images containing camo gear likely gives you improved likelihood of having one of your images included in top ranking image results.
- Outdoor labels are also overrepresented: wildlife, trees, plants, animals, etc. Images of hunters in camo, out in the wild, and with animals near them are disproportionately represented.
Looking closer at the distribution labels by keyword category can give use a deeper understanding of how the ranking images differ between similar keywords.
Here we see:
- For “turkey hunting gear” and “duck hunting gear,” having birds in your images seems very important, with the other keywords rarely including images with birds.
- Easy comparisons are possible with the interactive Tableau dashboards, giving you an “at a glance” understanding of what image distributions look like for an individual keyword vs. any other or all others. Below I highlighted just “duck hunting gear,” and you can see similar distribution of the most prevalent labels as the other keywords at the top. However, hugely overrepresented are “water bird,” “duck,” “bird,” “waders,” “hunting dog,” “hunting decoy,” etc., providing ample ideas for great images to include in the body of your content.
Ranking comparisons
Getting an intuition for the differences in top ranking (images ranking in the first 10 images for a keyword search) vs. bottom ranking (images ranking in the 41st to 50th positions) is also possible.
Here we can see that some labels seem preferred for top rankings. For instance:
- Clothing-related labels are much more common amongst the best ranking images.
- Animal-related labels are less common amongst the best ranking images but more common amongst the lower ranking images.
- Guns seem significantly more likely to appear in top ranking images.
By investigating trends in labels across your keywords, you can gain many interesting insights into the images most likely to rank for your particular niche. These insights will be different for any set of keywords, but a close examination of the results will yield more than a few actionable insights.
Not surprisingly, there are ways to go even deeper in your analysis with other artificial intelligence APIs. Let’s take a look at how we can further supplement our efforts.
An even deeper analysis for understanding
Deepai.org has an amazing suite of APIs that can be easily accessed to provide additional image labeling capabilities. One such API is “Image Captioning,” which is similar to Google’s image labeling, but instead of providing single labels, it provides descriptive labels, like “the man is holding a gun.”
We ran all of the same images as the Google label detection through this API and got some great additional detail for each image.
Just as with the label analysis, I broke up the caption distributions and analyzed their distributions by keyword and by overall frequency for all of the selected keywords. Then I compared top and bottom ranking images.
A final interesting finding
Google sometimes ranks YouTube video thumbnails in image search results. Below is an example I found in the hunting gear image searches.
It seems likely that at least some of Google’s understanding of why this thumbnail should rank for hunting gear comes from its image label detection. Though other factors, like having “hunting gear” in the title and coming from the NRA (high topical authority) certainly help, the fact that this thumbnail depicts many of the same labels as other top-ranking images must also play a role.
The lesson here is that the right video thumbnail choice can help that thumbnail to rank for competitive terms, so apply your learnings from doing image search result label and caption analysis to your video SEO strategy!
In the case of either video thumbnails or standard images, don’t overlook the ranking potential of the elements featured — it could make a difference in your SERP positions.